I am an engineer and researcher broadly interested in Probabilistic Machine Learning, Perception and
Geometry,
with a focus on Conditional Diffusion Models, Language Models Adaptation, Few-Shot Generation, and Hierarchical Variational
Inference.
|
Research
I am interested in the generalization and adaptation capabilities of hierarchical
generative models.
Large generative models trained on billions of data points demonstrate remarkable few-shot adaptation,
enabling them to tackle novel tasks with just a few examples.
However, these properties tend to manifest only at scale and are not present in smaller models,
which are commonly used in engineering and scientific discovery
where data is costly to gather and computational resources are limited.
My research aims to bridge the gap in adaptation capabilities between large and small generative models,
leveraging the tools of hierarchical inference and using the training dataset to encourage adaptation at inference time.
|
Preprints
|
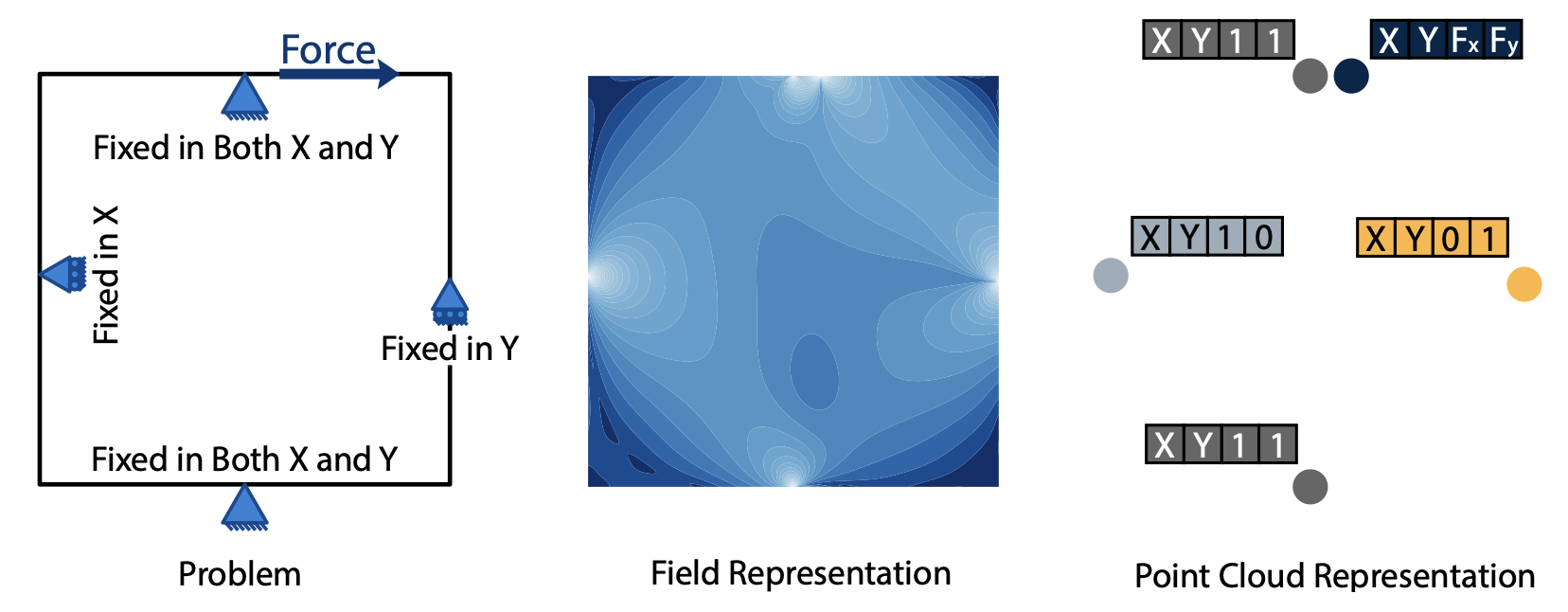 |
NITO: Neural Implicit Fields for Resolution-free Topology Optimization
Amin Heyrani Nobari,
Giorgio Giannone,
Lyle Regenwetter,
Faez
Ahmed
Preprint, arXiv, 2024
Topology optimization is a critical task in engineering design, where the goal is to optimally distribute material in a given space for maximum performance.
We introduce Neural Implicit Topology Optimization (NITO), a novel approach to accelerate topology optimization problems using deep learning.
NITO stands out as one of the first frameworks to offer a resolution-free and domain-agnostic solution in deep learning-based topology optimization.
NITO synthesizes structures with up to seven times better structural efficiency compared to SOTA diffusion models and does so in a tenth of the time.
In the NITO framework, we introduce a novel method, the Boundary Point Order-Invariant MLP (BPOM), to represent boundary conditions in a sparse and domain-agnostic manner,
moving away from expensive simulation-based approaches.
|
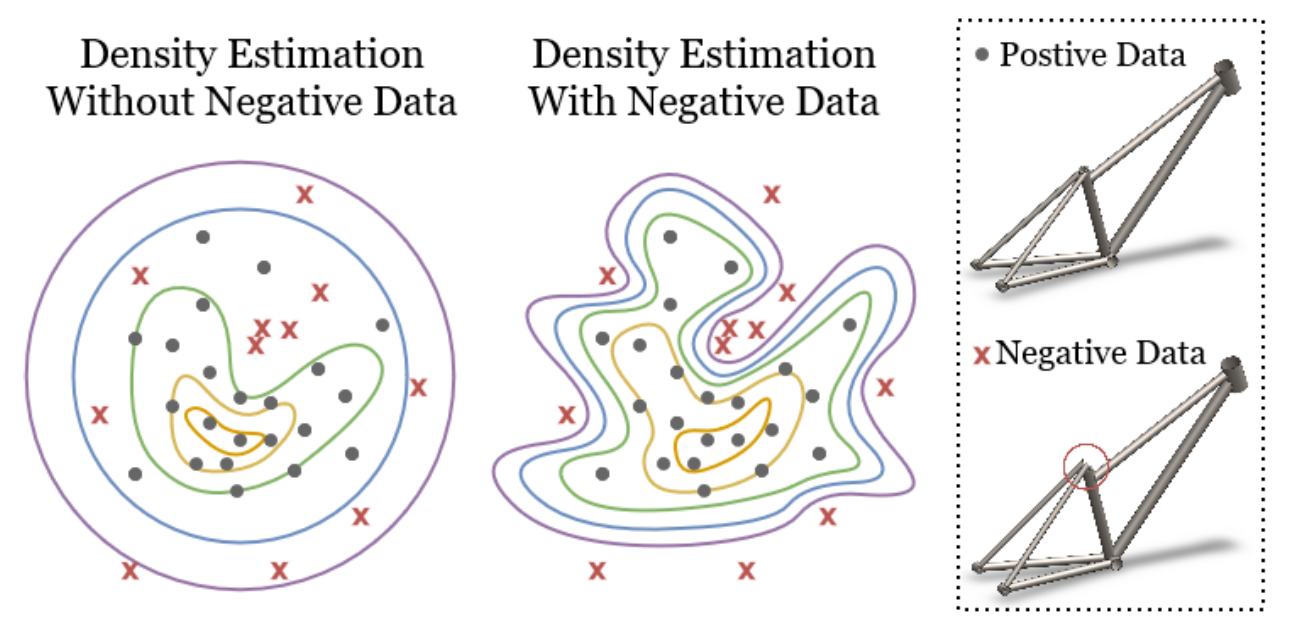 |
Constraining Generative Models for Engineering Design with Negative Data
Lyle Regenwetter*,
Giorgio Giannone*,
Akash Srivastava,
Dan
Gutfreund,
Faez
Ahmed
Preprint, arXiv, 2024
Generative models have recently achieved remarkable success and widespread adoption in society, yet they still often struggle to generate realistic and accurate outputs.
This challenge extends beyond language and vision into fields like engineering design, where safety-critical engineering standards and
non-negotiable physical laws tightly constrain what outputs are considered acceptable.
In this work, we introduce two approaches to guide models toward constraint-satisfying outputs using negative data -- examples of what to avoid.
|
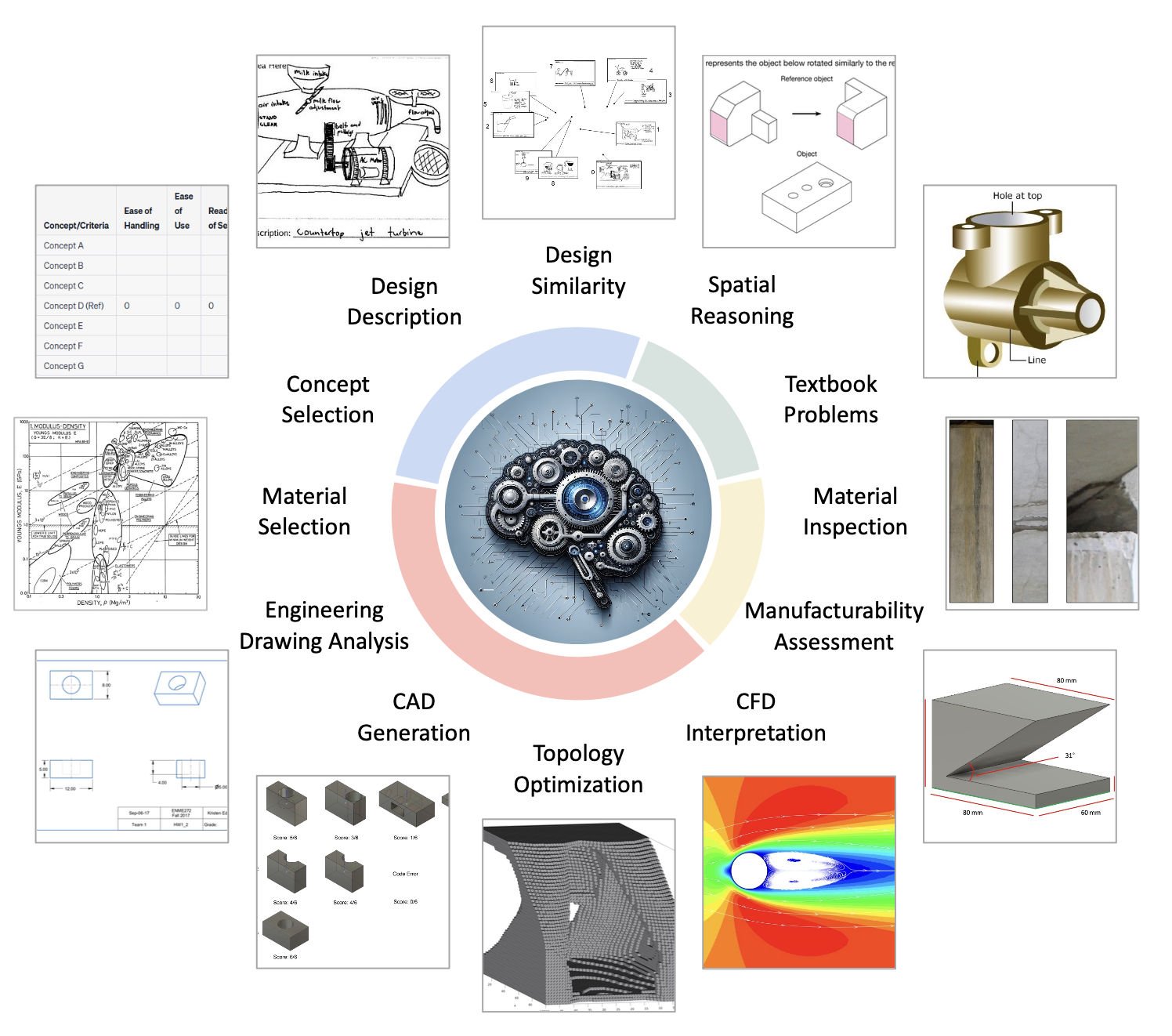 |
From Concept to Manufacturing: Evaluating Vision-Language Models for Engineering Design
Cyril Picard*,
Kristen M. Edwards*,
Anna C. Doris,
Brandon Man,
Giorgio Giannone,
Md Ferdous Alam,
Faez Ahmed
Under Review, 2024
Engineering Design is undergoing a transformative shift with the advent of AI, marking a new era in how we approach product, system, and service planning.
Large language models have demonstrated impressive capabilities in enabling this shift.
Yet, with text as their only input modality, they cannot leverage the large body of visual artifacts that engineers have used for centuries and are accustomed to.
This gap is addressed with the release of multimodal vision language models, such as GPT-4V, enabling AI to impact many more types of tasks.
In light of these advancements, this paper presents a comprehensive evaluation of GPT-4V, a vision language model, across a wide spectrum of engineering design tasks,
categorized into four main areas: Conceptual Design, System-Level and Detailed Design, Manufacturing and Inspection, and Engineering Education Tasks.
|
Publications
|
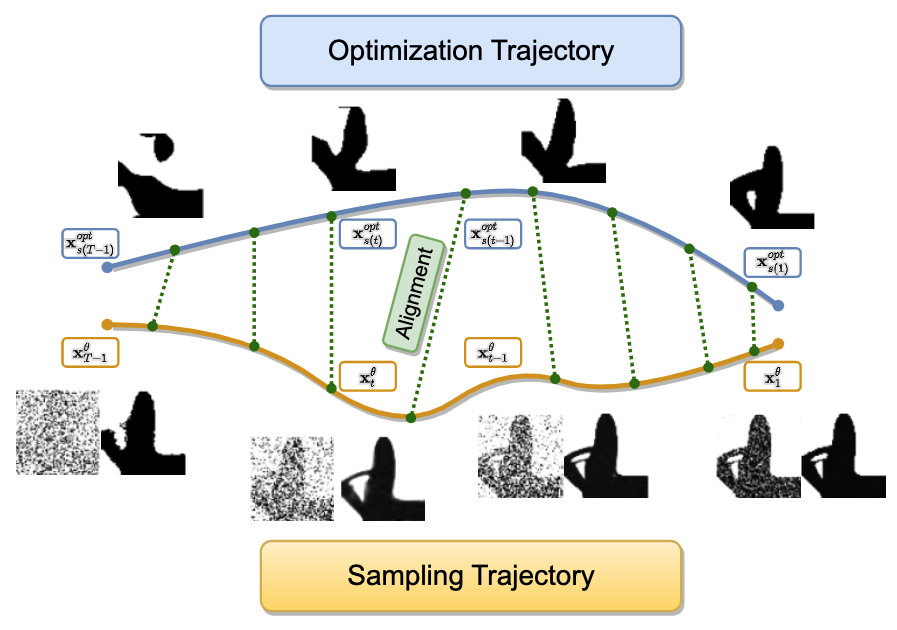 |
Aligning Optimization Trajectories with Diffusion Models for
Constrained Design Generation
Giorgio Giannone,
Akash Srivastava,
Ole Winther,
Faez
Ahmed
Neural Information Processing Systems, NeurIPS, 2023
Generative models have had a profound impact on vision and language, paving the
way for a new era of multimodal generative applications.
While these successes have inspired researchers to explore using generative
models in science and engineering to accelerate the design process and reduce
the reliance on iterative optimization, challenges remain.
Specifically, engineering optimization methods based on physics still outperform
generative models when dealing with constrained environments where data is
scarce and precision is paramount.
To address these challenges, we introduce Diffusion Optimization Models (DOM)
and Trajectory Alignment (TA),
a learning framework that demonstrates the efficacy of aligning the sampling
trajectory of diffusion models with the optimization trajectory derived from
traditional physics-based methods.
|
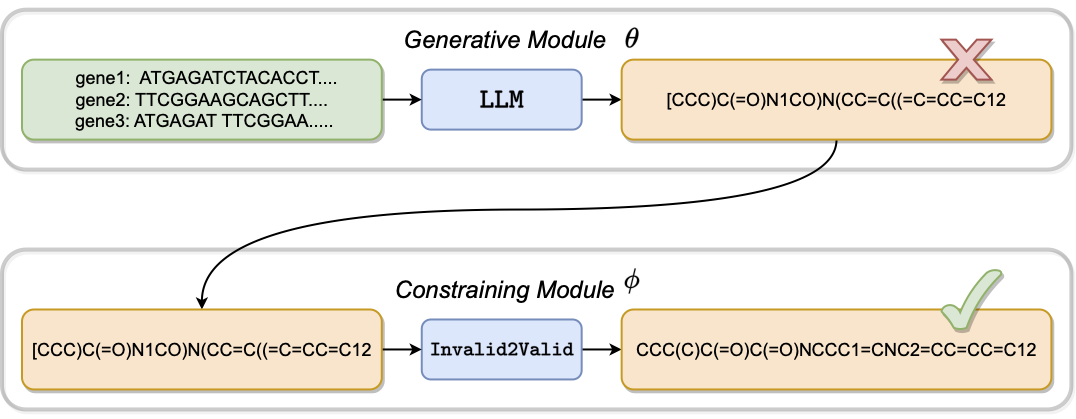 |
Improving Precision in Language Models Learning from Invalid
Samples
Niels Jakob Larsen*,
Giorgio Giannone*,
Ole Winther,
Kai
Blin
Generative AI and Biology Workshop, NeurIPS, 2023
Language Models are powerful generative tools capable of learning intricate patterns
from vast amounts of unstructured data. Nevertheless, in domains that demand
precision, such as science and engineering, the primary objective is to obtain an
exact and accurate answer. Precision takes precedence in these contexts. In
specialized tasks like chemical compound generation, the emphasis is on output
accuracy rather than response diversity. Traditional self-refinement methods are
ineffective for such domain-specific input/output pairs, unlike general language
tasks. In this study, we introduce invalid2valid, a powerful and general
post-processing mechanism that can significantly enhance precision in language
models for input/output tasks spanning different domains and specialized
applications.
|
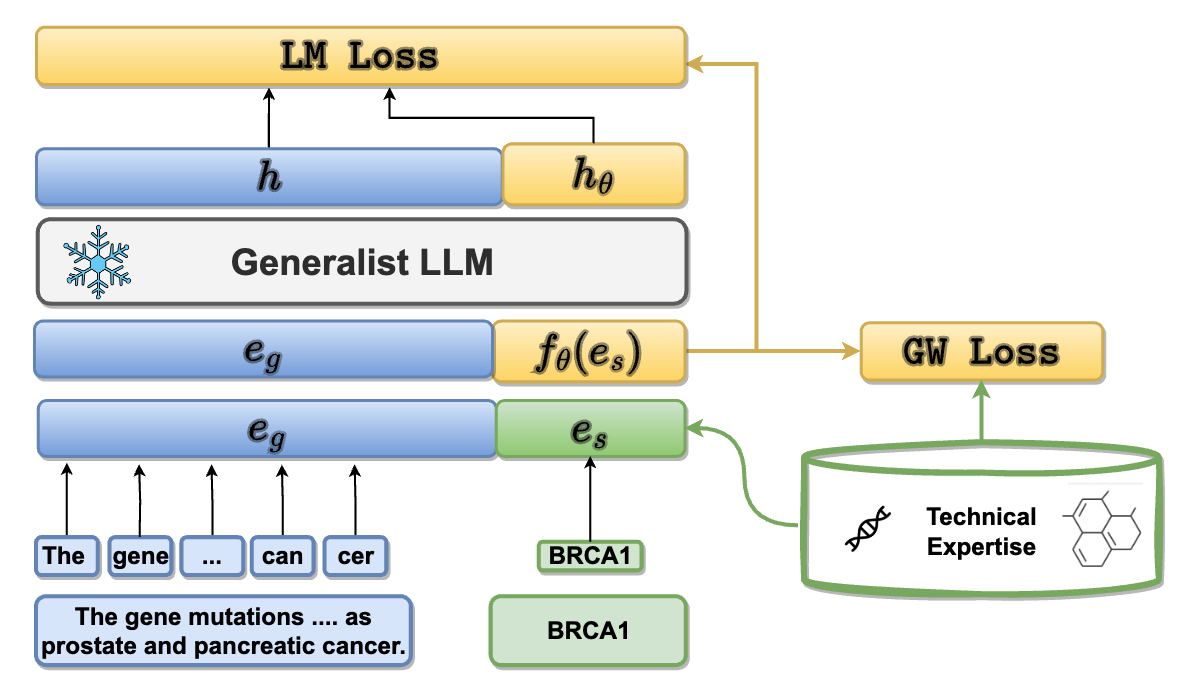 |
Enhancing Language Models for Technical Domains with Dynamic
Token Injection
Giorgio Giannone,
Neil Tenenholtz,
James Hall,
Nicolo Fusi,
David Alvarez-Melis
Generative AI and Biology Workshop, NeurIPS, 2023
Large language models (LLMs) are rapidly advancing the frontier of natural language
understanding and generation. Their generalist nature, while adept at handling a
wide range of tasks, often lacks the depth and precision required by highly
specialized and rapidly evolving technical domains, such as genomics and engineering
design. Fine-tuning these models for specific domains can be effective but requires
large amounts of data and compromises their general reasoning capabilities. In this
work, we introduce a scalable method to infuse specialized knowledge into generalist
language models by dynamically extending their vocabulary with specialist tokens. By
using a lightweight functional mapping on an extended vocabulary and adjusting the
logit distribution, we enable the model to grasp domain-specific nuances.
|
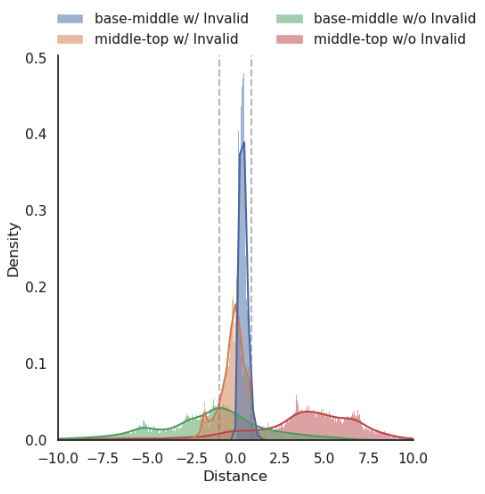 |
Learning from Invalid Data: On Constraint Satisfaction in
Generative Models
Giorgio Giannone*,
Lyle Regenwetter*,
Akash Srivastava*,
Dan
Gutfreund,
Faez
Ahmed
Diffusion Models Workshop, NeurIPS, 2023
Generative models have demonstrated impressive results in vision, language, and
speech.
However, even with massive datasets, they struggle with precision, generating
physically invalid or factually incorrect data.
This is particularly problematic when the generated data must satisfy
constraints, for example, to meet product specifications in engineering design
or to adhere to the laws of physics in a natural scene.
To improve precision while preserving diversity and fidelity, we propose a novel
training mechanism that leverages datasets of constraint-violating data points,
which we consider invalid.
|
|
Unifying Molecular and Textual Representations via Multi-task
Language Modelling
Dimitrios Christofidellis*,
Giorgio Giannone*,
Jannis
Born,
Ole Winther,
Teodoro
Laino,
Matteo
Manica
International Conference on Machine Learning, ICML, 2023
The recent advances in neural language models have also been successfully
applied to the field of chemistry,
offering generative solutions for classical problems in molecular design and
synthesis planning. These new methods have the potential to optimize laboratory
operations and fuel
a new era of data-driven automation in scientific discovery. However,
specialized models are still typically required for each task, leading to the
need for problem-specific
fine-tuning and neglecting task interrelations. Here, we propose a
multi-domain, multi-task language model to solve a wide range of tasks in both
the chemical and natural
language domains. By leveraging multi-task learning, our model can handle
chemical and natural language concurrently, without requiring expensive
pre-training on single
domains or task-specific models.
|
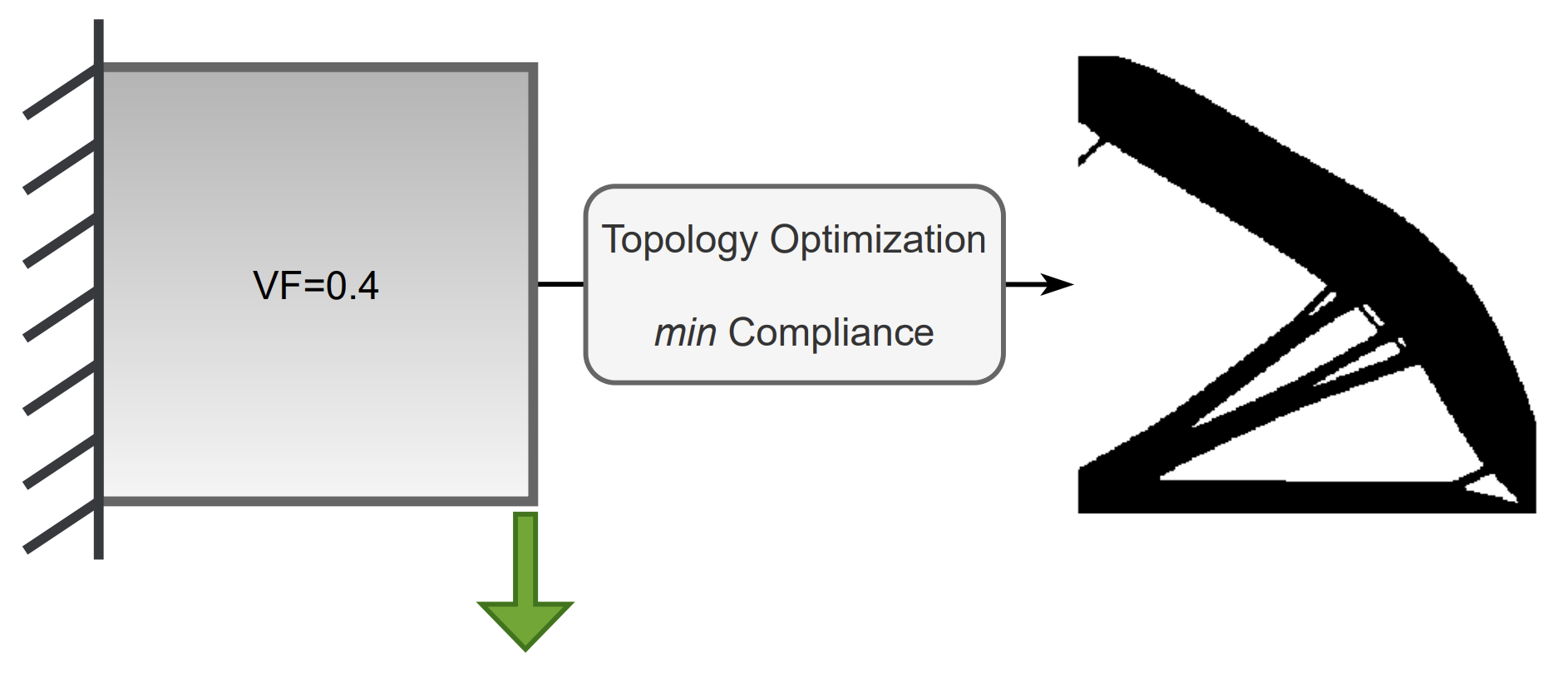 |
Diffusing the Optimal Topology: A Generative Optimization
Approach
Giorgio Giannone,
Faez
Ahmed
International Design Engineering Technical Conferences, IDETC, 2023
Topology Optimization seeks to find the best design that satisfies a set of
constraints while maximizing system performance.
Traditional iterative optimization methods like SIMP can be computationally
expensive and get stuck in local minima,
limiting their applicability to complex or large-scale problems.
Recently, deep generative models, such as Generative Adversarial
Networks and Diffusion Models,
conditioned on constraints and physics fields have shown promise, but they
require extensive pre-processing and surrogate models for improving performance.
To address these issues, we propose a Generative Optimization method that
integrates classic optimization like SIMP as a refining mechanism for the
topology generated by a deep generative model.
|
|
Accelerating material design with the generative toolkit for
scientific discovery
Manica & the GT4SD
Team (Core Contributor)
Nature npj Computational Materials, 2023
With the growing availability of data within various scientific domains,
generative models hold enormous potential to accelerate scientific discovery.
They harness powerful representations learned from datasets to speed up the
formulation
of novel hypotheses with the potential to impact material discovery broadly.
We present the Generative Toolkit for Scientific Discovery (GT4SD).
This extensible open-source library enables scientists, developers,
and researchers to train and use state-of-the-art generative models
to accelerate scientific discovery focused on organic material design.
|
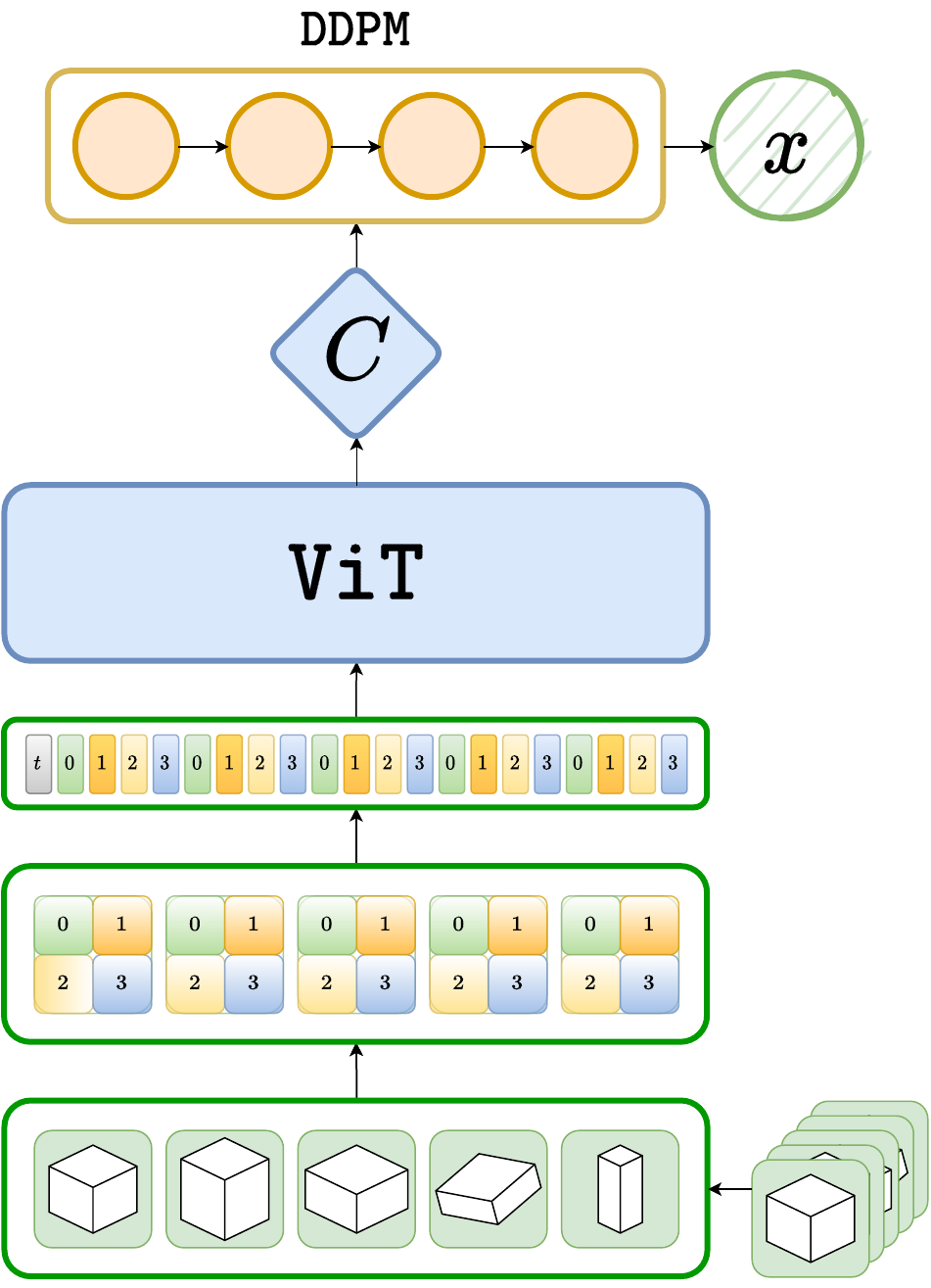 |
Few-Shot Diffusion Models
Giorgio Giannone,
Didrik Nielsen,
Ole Winther
Score-Based Methods Workshop, NeurIPS, 2022
Denoising diffusion probabilistic models (DDPM) are powerful hierarchical latent
variable models with remarkable sample generation quality and training
stability.
These properties can be attributed to parameter sharing in the generative
hierarchy, as well as a parameter-free diffusion-based inference procedure.
In this paper, we present Few-Shot Diffusion Models (FSDM), a framework for
few-shot generation leveraging conditional DDPMs.
FSDMs are trained to adapt the generative process conditioned on a small set of
images from a given class by aggregating image patch information using a
set-based Vision Transformer (ViT).
At test time, the model is able to generate samples from previously unseen
classes conditioned on as few as 5 samples from that class.
We empirically show that FSDM can perform few-shot generation and transfer to
new datasets taking full advantage of the conditional DDPM.
|
|
SCHA-VAE: Hierarchical Context Aggregation for
Few-Shot Generation
Giorgio Giannone,
Ole Winther
International Conference on Machine Learning, ICML, 2022
A few-shot generative model should be able to generate data from a novel
distribution by only observing a limited set of examples.
In few-shot learning the model is trained on data from many sets from
distributions sharing some underlying properties such
as sets of characters from different alphabets or objects from different
categories.
We extend current latent variable models for sets to a fully hierarchical
approach with an attention-based point to
set-level aggregation and call our method SCHA-VAE for
Set-Context-Hierarchical-Aggregation Variational Autoencoder.
We explore likelihood-based model comparison, iterative data sampling, and
adaptation-free out-of-distribution generalization.
|
|
Just Mix Once: Worst-group Generalization by Group
Interpolation
Giorgio Giannone,
Serhii
Havrylov,
Jordan Massiah,
Emine
Yilmaz,
Yunlong Jiao
Distribution Shifts Workshop, NeurIPS, 2021
Advances in deep learning theory have revealed how average generalization relies
on superficial patterns in data.
The consequences are brittle models with poor performance with shift in group
distribution at test time.
When group annotation is available, we can use robust optimization tools to
tackle the problem.
However, identification and annotation are time-consuming, especially on large
datasets.
A recent line of work leverages self-supervision and oversampling to improve
generalization on minority groups
without group annotation. We propose to unify and generalize these approaches
using a class-conditional variant
of mixup tailored for worst-group generalization. Our approach, Just Mix Once
(JM1),
interpolates samples during learning, augmenting the training distribution with
a continuous mixture of groups.
|
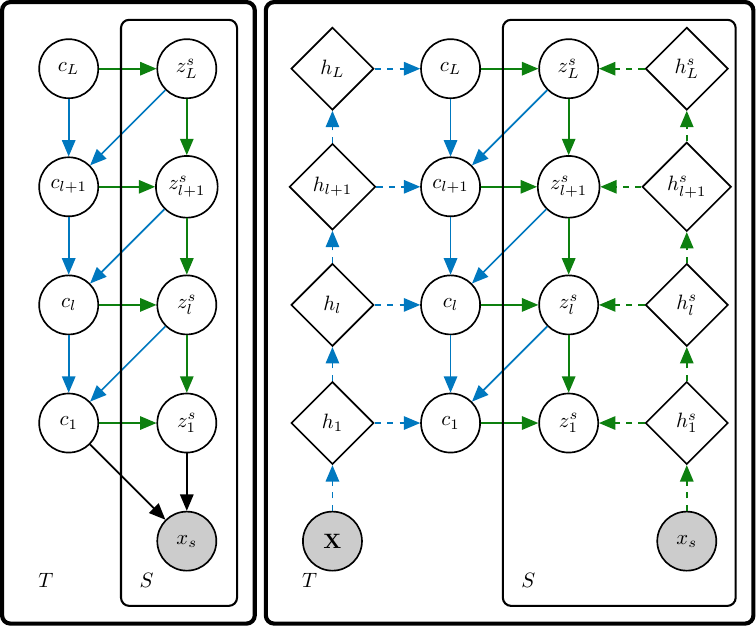 |
Hierarchical Few-Shot Generative Models
Giorgio Giannone,
Ole Winther
Meta-Learning Workshop, NeurIPS, 2021
A few-shot generative model should be able to generate data from a distribution
by only observing a limited set of examples. In few-shot learning the model is
trained on data from many sets from different distributions sharing some
underlying
properties such as sets of characters from different alphabets or sets of images
of different type objects. We study a latent variables approach that extends the
Neural Statistician to a fully hierarchical approach with an attention-based
point to set-level aggregation. We extend the previous work to iterative data
sampling,
likelihood-based model comparison, and adaptation-free out of distribution
generalization.
|
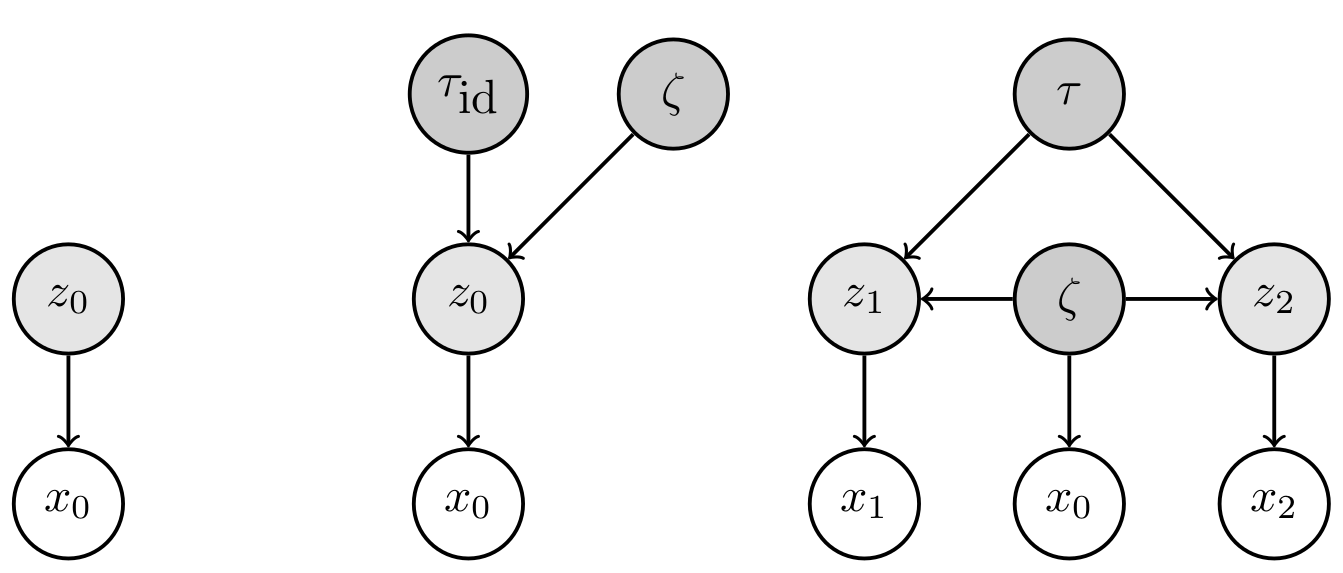 |
Transformation-aware Variational Autoencoder
Giorgio Giannone,
Saeed
Saremi,
Jonathan Masci,
Christian Osendorfer
Technical Report, 2020
We extend the framework of variational autoencoders to represent transformations
explicitly in the latent space. This is achieved in the form of a generative
model
structured such that the group of transformations
that act in the input space is instead represented by latent variables
which are linear operators that only act in the latent space.
In the family of hierarchical graphical models that emerges,
the latent space is populated by higher order objects which are inferred
jointly with the latent representations they act on.
|
|
Real-time Classification from Short Event-Camera Streams using
Input-filtering Neural ODEs
Giorgio Giannone,
Asha Anoosheh,
Alessio Quaglino,
Pierluca D'Oro,
Marco
Gallieri,
Jonathan Masci
Interpretable Inductive Biases
and Physically Structured Learning Workshop, NeurIPS, 2020
Event-based cameras are novel, efficient sensors inspired by the human vision
system,
generating an asynchronous, pixel-wise stream of data.
Learning from such data is generally performed through heavy preprocessing and
event integration into images.
This requires buffering of possibly long sequences and can limit the response
time of the inference system.
In this work, we instead propose to directly use events from a DVS camera,
a stream of intensity changes and their spatial coordinates.
This sequence is used as the input for a novel asynchronous RNN-like
architecture,
the Input-filtering Neural ODEs.
|
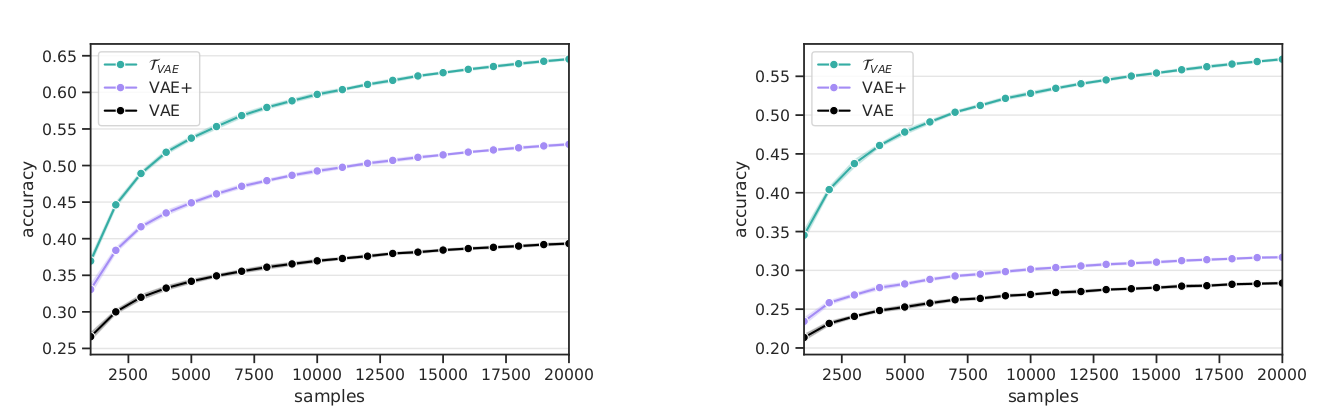 |
No Representation without Transformation
Giorgio Giannone,
Jonathan Masci,
Christian Osendorfer
Bayesian Deep Learning and Perception as Generative Reasoning Workshops,
NeurIPS , 2019
We propose to extend Latent Variable Models with a simple idea:
learn to encode not only samples but also transformations of such samples.
This means that the latent space is not only populated by embeddings
but also by higher order objects that map between these embeddings.
We show how a hierarchical graphical model can be utilized to enforce
desirable algebraic properties of such latent mappings.
|
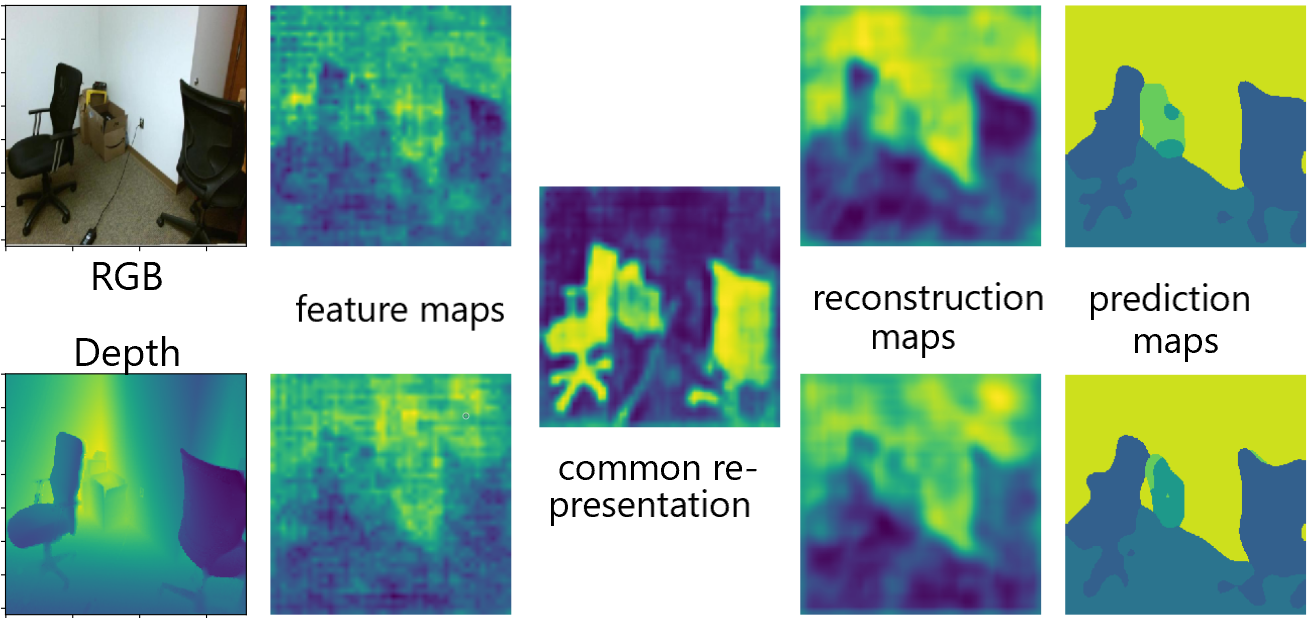 |
Learning Common Representation from RGB and Depth Images
Giorgio Giannone,
Boris Chidlovskii
Multimodal Learning and Applications Workshop, CVPR, 2019
We propose a new deep learning architecture for the tasks of semantic
segmentation
and depth prediction from RGB-D images.
We revise the state of art based on the RGB and depth feature fusion,
where both modalities are assumed to be available at train and test time.
We propose a new architecture where the feature fusion is replaced with a common
deep representation.
Combined with an encoder-decoder type of the network, the architecture can
jointly learn models
for semantic segmentation and depth estimation based on their common
representation.
|
Datasets
|
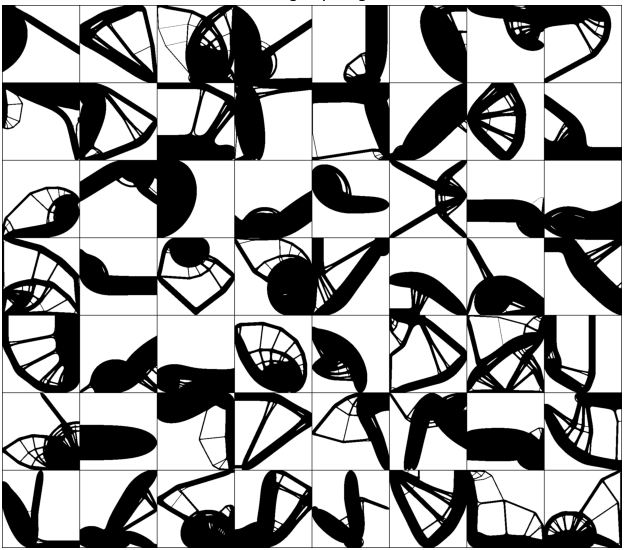 |
2d Topology Optimization
We built a dataset of optimized topologies and intermediate optimization steps at
low-resolution (64x64) and
high-resolution (256x256) with constraints.
- 50K low-resolution optimized topologies.
- 60K high-resolution optimizer topologies.
- 250K low-resolution intermediate steps.
- 300K high-resolution intermediate steps.
|
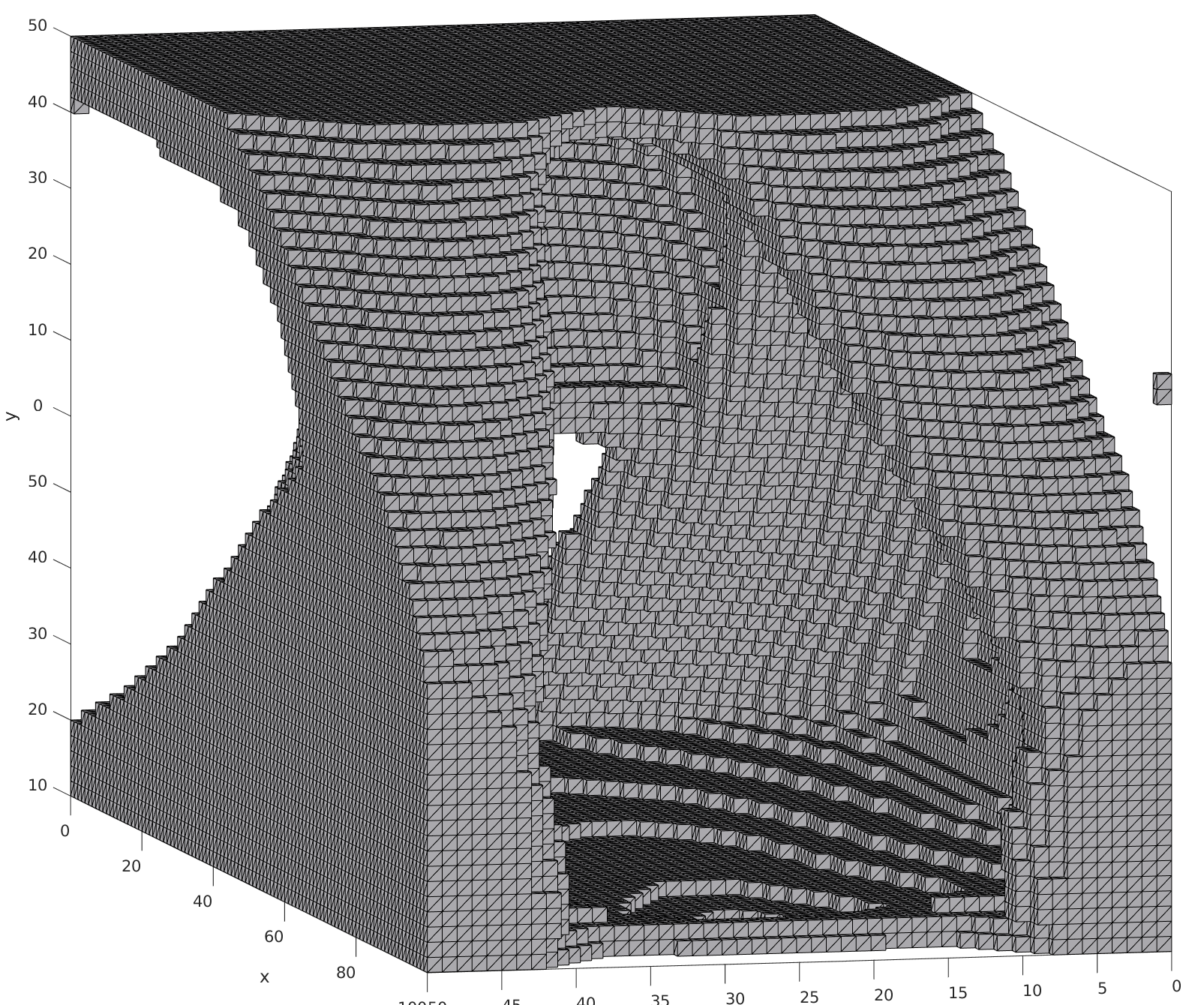 |
3d Topology Optimization
We built a multifidelity dataset of 300K optimized topologies with constraints.
- 150K beams.
- 100K plates.
- 50K l-shapes.
|
Theses
|
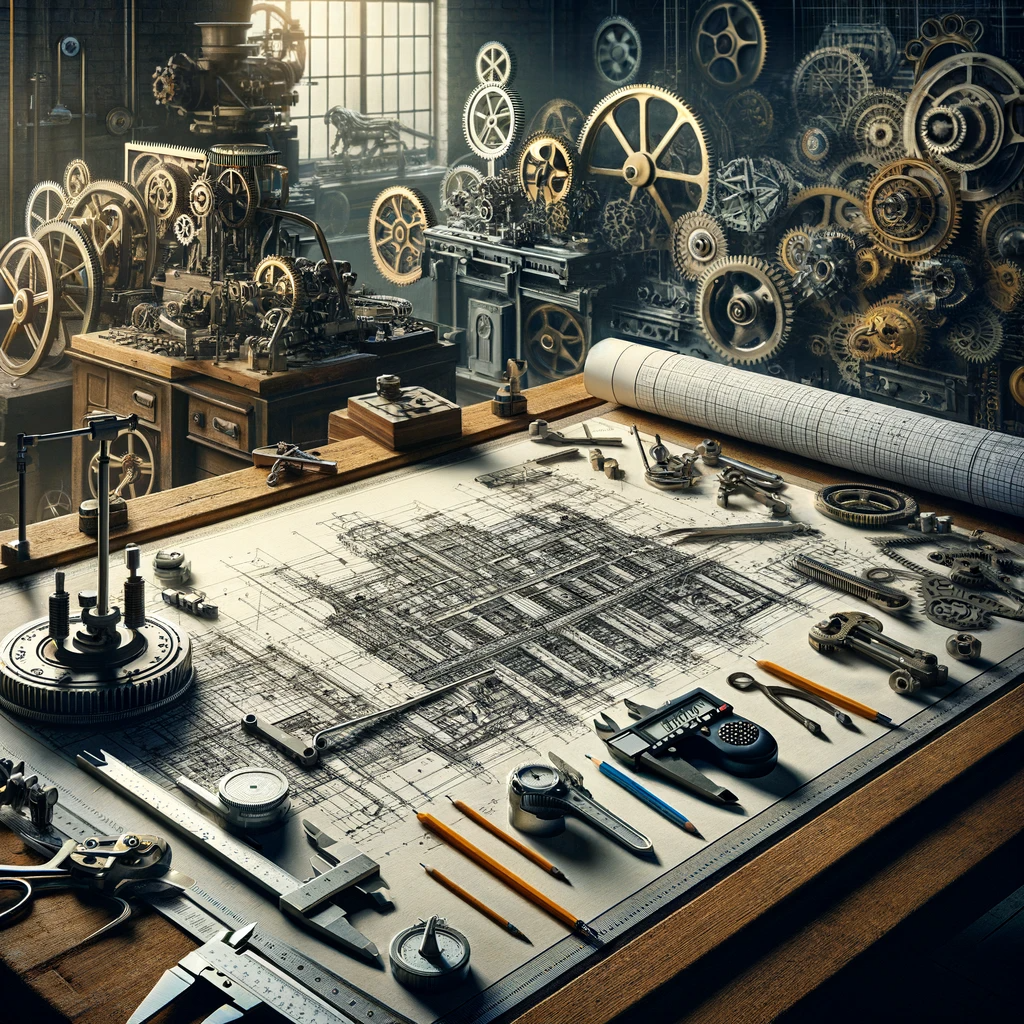 |
Few-Shot Generative Models: Learning Generative Models from Limited Data
Giorgio Giannone
PhD's Thesis, Machine Learning, Technical University of Denmark, 2023
|
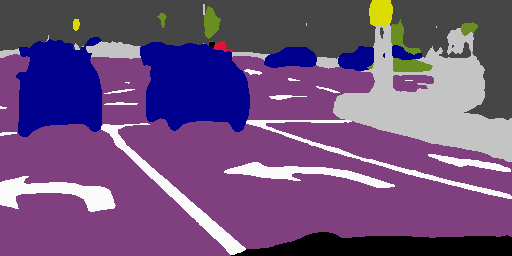 |
Learning Common Representation for Scene Understanding
Giorgio Giannone
Master's Thesis, Data Science, Sapienza University of Rome, 2018
|
|
Bubble Dynamics in Turbulent Shear Flows
Giorgio Giannone
Master's Thesis, Mechanical Engineering, Sapienza University of Rome,
2016
|
|